How Walmart Puts AI and Machine Learning into Play
Our cohorts’ takeaways from top retail/tech AI expert
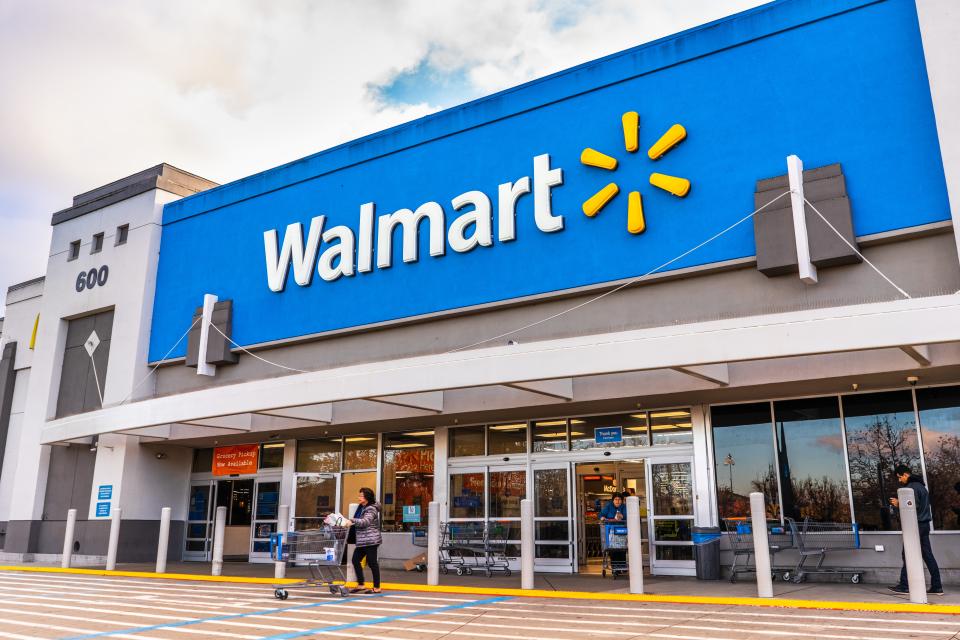
With its potential to automate operations, enhance customer experience and make data-driven decisions, it’s no secret that artificial intelligence (AI) is radically changing how businesses operate.
My cohort of Master of Science in Business Analytics (MSBA) students tackle projects and problem-solving using AI tools, and we learn how we can bring those skills to our careers.
The UC Davis MSBA program often invites top executives to exclusive events where we have the opportunity to hear insights from industry leaders and the latest trends.
Walmart’s AI Strategy
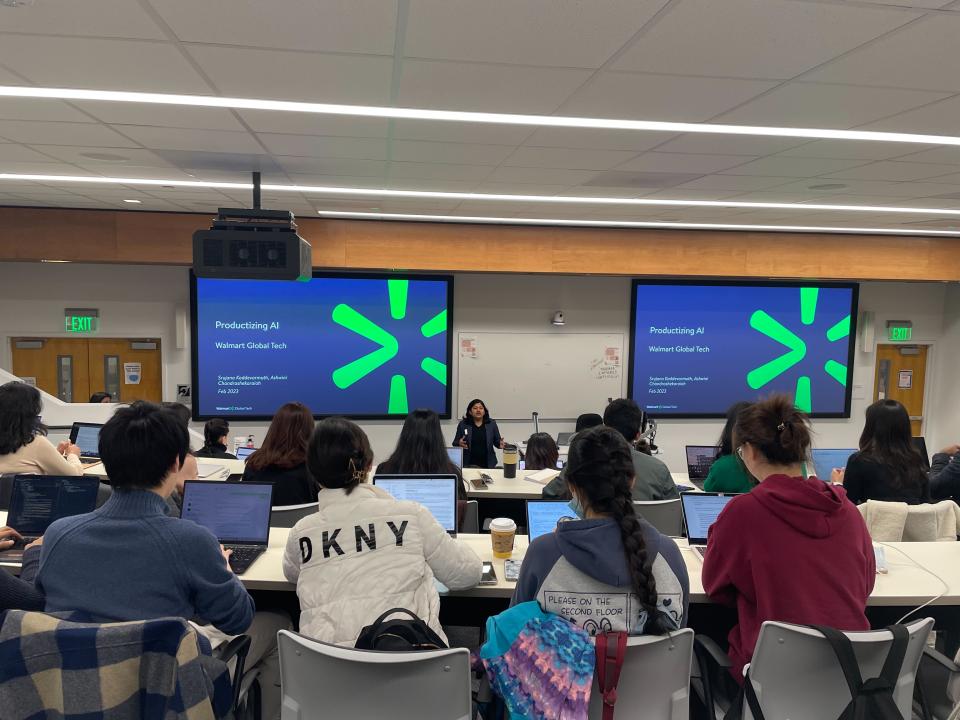
As part of the program’s Winter Path to Career Success Series, we met Srujana Kaddevarmuth, senior director of data and machine learning center of excellence at Walmart. She is a leading AI expert and has made major contributions to its development. She shared about Walmart's AI strategy and how it has been productized.
Kaddevarmuth focused her talk on three main areas and their learning outcomes:
- Recommendation Algorithms: She covered various recommendation algorithms available and used in the retail/technology industry, how did these algorithms evolve and the pros and cons.
Our Learning Outcomes: A greater understanding of evolutions and trends of recommender systems, the real-life constraints and workarounds needed for successful deployment.
- Technology Stack: How to deploy recommender systems at scale. Underlying technology stack and evolution of this tech stack. Glimpse into practical considerations of computational needs, functionalities and architectural efficiencies needed to deploy these systems in the industry.
Our Learning Outcomes: A greater understanding of the importance of technology stack, design and architectural considerations to deploy data science algorithms in real life/industry.
- Productizing AI: How to develop a high throughput product (recommendation engine), benefits of productizing algorithms and modularizing codes risk associated with productizing algorithms and ethical and regulatory considerations for effective governance of these products to mitigate unintended consequences.
Our Learning Outcomes: A better understanding of the process of productizing AI, associated benefits and risks.
She first discussed the evolution of Applied Recommender Systems at Walmart.
She highlighted how AI has played a crucial role in Walmart's success by improving inventory management, detecting suspicious activity and enhancing customer experience.
She explained that Walmart has been using collaborative filtering and content-based filtering techniques to personalize the customer experience. Collaborative filtering analyzes the interaction matrix between the user and the item to create user profiles and history. On the other hand, content-based filtering analyzes item attributes to create related item profiles.
Walmart also uses deep learning-based recommender systems such as convolutional neural networks (CNNs) and recurrent neural networks (RNNs), which can analyze image and text datasets, respectively.
Ethical Challenges of AI
Kaddevarmuth also discussed the challenges associated with AI systems, including the risk of bias and the filter bubble. Walmart has been building ethical AI systems that are representative of the data and inclusive of diverse users. Additionally, the shift to the digital economy has significantly impacted customer behavior, and Walmart has been leveraging AI to tap into this change.
Kaddevarmuth also addressed productization, the process of making AI into a sellable product. She described the process of developing AI products, going from the proof-of-concept stage through the deployment stage.
She emphasized how crucial it is for product managers, legal teams, and other stakeholders to work collaboratively to define, simplify, communicate, expand, execute and monitor AI solutions.
Kaddevarmuth also spoke on the importance of building an AI architecture that is constantly fed with new data to maintain its accuracy. She emphasized the need for data wrappers to mitigate model deviation and high bias in accuracy. Additionally, she discussed the risks associated with productizing AI, including institutionalization bias and the need for efficient use of resources.
Finally, Kaddevarmuth explained how Walmart has been mitigating these risks by focusing on efficiency, mitigating bias and using a cross-functional approach to productizing AI.
The event provided us with insightful discussions on the evolution of Applied Recommender Systems and Walmart's approach to AI productization serves as a valuable lesson for businesses looking to integrate AI into their operations.