Small Data Tells a Big Story
Big Data is overblown, bigger problems lack data
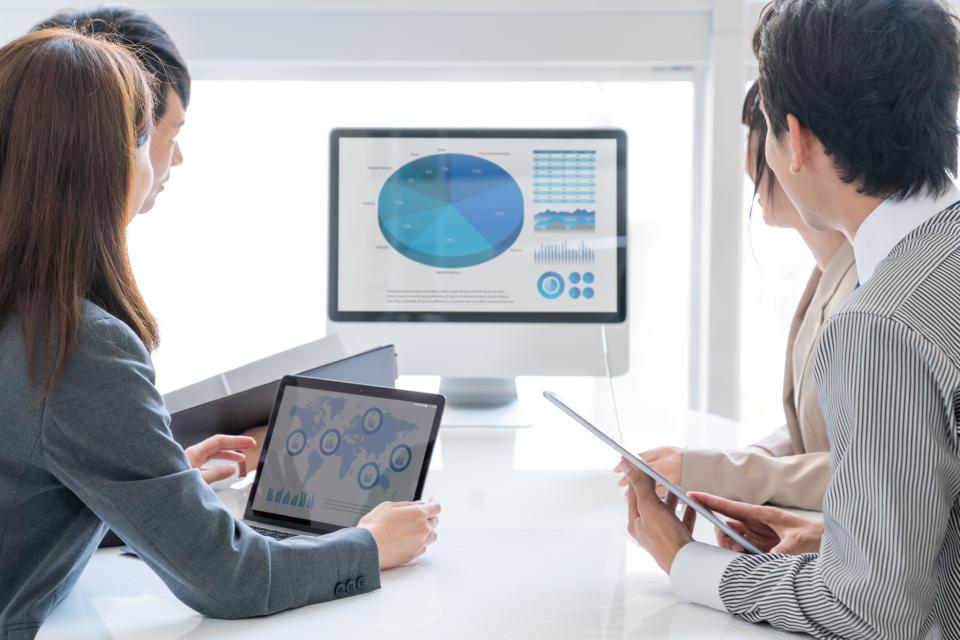
Everyone is talking about big data today. While there are many applications and opportunities to harness big data to disrupt industries and revolutionize company operations, the conventional wisdom proclaims you should feel ashamed if you don’t solve a problem using big data. That’s not the truth.
Small Data Conundrum
Many problems data analysts encounter are ‘small data’ problems. In many instances, we don’t have enough data to perform statistical analysis, build models and apply complicated algorithms.
This is especially true in fields such as medicine, sociology and farming. Through my MSBA team’s work in these fields, we’ve discovered it’s near impossible to collect ‘big’ enough data to solve the problems due to the cost and feasibility.
So what should we do?
There Are Two Main Solutions
Creating simpler models and applying domain experience is key to solving small-data problems in the real world. — MSBA student Xinyu Zhang
Solution 1: Choose Simpler Models
Overfitting is always a big concern when we train models on small data. But by building simpler models, we can avoid overfitting and poor generalization and we can get to our solutions faster.
In our MSBA practicum project, we focused on linear models and assembled them using bagging, stacking or boosting methods. We think we could even build simpler models to make our conclusions and models easier to explain and more robust, which can help our sponsor client, Bowles Farming, to make better decisions in the future.
Solution 2: Apply Domain Expertise
In practice, domain expertise or industry experience plays a crucial part in handling sophisticated problems. In the small data scenario, domain expertise is even more important because it’s a great supplement to data analysis. In our practicum project, we should definitely include farming knowledge and experience in our analysis. Actually, we rely most on the domain knowledge and data only provides some part of the analysis.
Business Storytelling at Work
Building models is not challenging at all, even with small data. What always gets in the way is how to present the findings through engaging stories.
For the most part, our clients, customers and partners are probably not data experts. They might struggle to see the solutions through the data forest. We need to adjust ourselves to an environment where most people are not data-driven or data-informed.
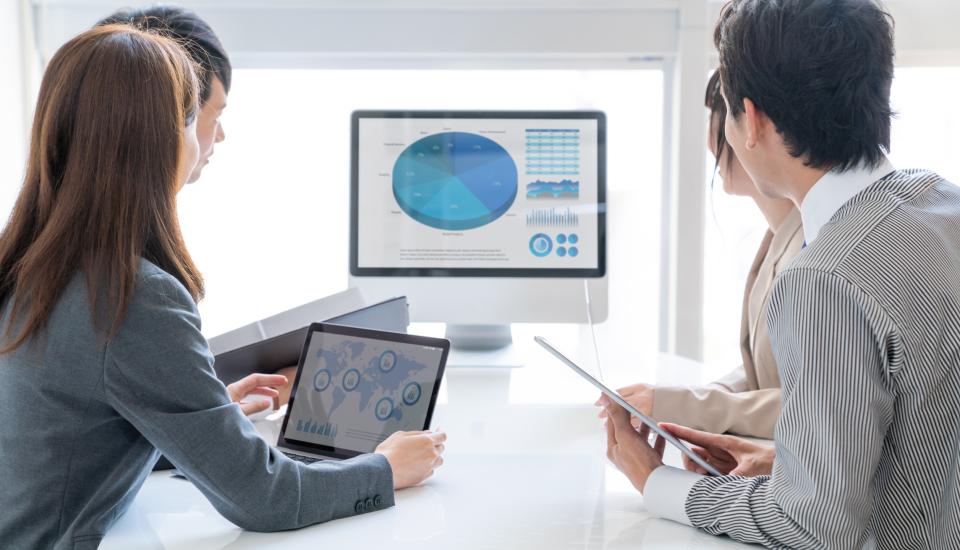
As data becomes more important to corporations and remains tough to decipher, it’s important to know that data visualization is the key to storytelling. An interactive visualization conveys more information than words. Word clouds, Facet Grids and maps are usually more helpful in presenting key conclusions and findings to stakeholders. Moreover, a good story structure also provides and requires strong support.
Incorporating a striking introduction, clean headings and practical conclusions makes any story more engaging and persuasive. In our practicum project, we utilize data visualizations to construct a coherent story that helps us communicate our thoughts to our client.
To sum up, small data problems are more common in the real world, and we need to adjust our data tactics to incorporate this fact. By telling strong stories with data visualizations, we can deliver solutions that create an impact on decision making in an organization.
We have learned this first-hand by honing our analytical skills in small data during our practicum project, and we’ve improved our competencies and performances for the future workplace.