Capturing Competitive Advantage With AI
Carrie Beam, MSBA Director of Analytics Projects
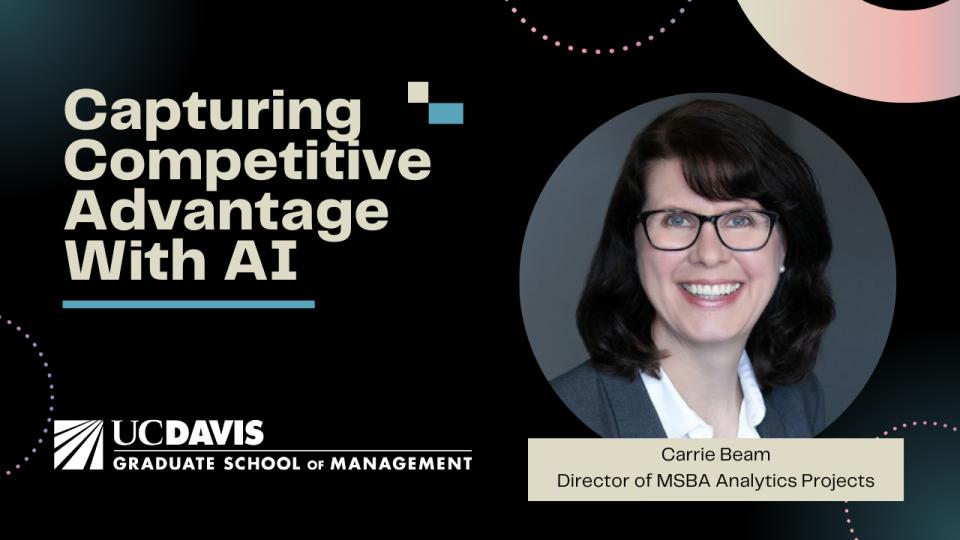
Carrie Beam, director of MSBA analytics projects at UC Davis, shared cutting-edge insights on data analytics and AI during the "Capturing Competitive Advantage with AI" webinar, hosted by Modern Ideas for Business.
She highlighted real-world projects from the UC Davis MSBA Analytics program that have helped industry partners gain a competitive edge through predictive analytics, machine learning, and market research.
In her webinar, Beam covers:
- How AI works, including its strengths and weaknesses
- Jobs most impacted by AI, highlighting which will benefit and which may face challenges
- A checklist for evaluating statements of work when hiring analytics professionals
- Common reasons analytics projects fail and strategies to prevent them
- Opportunities for companies to partner with UC Davis through student practicum projects
With her expertise, Beam also emphasized how the next generation of data professionals is helping business leaders leverage data and analytical models to transform their organizations.
Transcript
Dave Cowan, founder and CEO of Silicon Valley Sales Group
Good morning, everyone. I am Dave Cowan, founder and CEO of Silicon Valley Sales Group, a Sandler sales and management training company, enabling individuals, professional service providers, and businesses across a range of industries to achieve their revenue and growth goals. I am one of the organizers of Modern Ideas for Business, and I'll be your moderator today.
Our mission at Modern Ideas for Business is to enable business leaders to solve the multifaceted challenges they face while finding opportunities presented by changes in the economy, political and legal landscapes, and social trends in the markets they serve. We strive to empower business leaders with the knowledge, skills, networks, and actionable insights necessary to navigate the complexities of the modern business world and achieve sustainable business success.
The other organizers of Modern Ideas for Business are Ed Correa, founder and president of Segecin Technologies, a global leader in cybersecurity solutions and digital risk management; Paul Duran, a commercial banker for over 25 years and now an expert loan consultant, serving small and medium-sized businesses looking for methods and means to fund their growth; and Jaco Grobala, CEO of Broadvision Marketing, developing and executing inbound marketing strategies that enable clients to consistently create more sales-ready prospects.
We started Modern Ideas for Business with the goal of creating an interactive forum where business leaders can learn from experts in a variety of fields, ask questions, and share their thoughts and opinions on the topic of the day. During today's webinar, feel free to come off mute and ask questions or offer your thoughts, ideas, and opinions. We will also be monitoring chat if you want to ask questions there.
So, with that, I’m happy to introduce our speaker, Dr. Carrie Beam. Carrie graduated from Princeton University with degrees in civil engineering and operations research. She then earned her PhD at the University of California, Berkeley, in industrial engineering and operations research, which launched a decades-long career of university teaching and analytics consulting.
Carrie is now director of the Master of Science in Business Analytics program at the University of California at Davis, one of the top programs of its kind in the world. Through the MSBA program, Carrie and her colleagues develop high-performance professionals who can create business value from data and analytical models. As she’ll explain today, you can connect your company to this highly ranked program by becoming a sponsor of an MSBA practicum project.
Carrie is uniquely qualified to share her thoughts on the current state of artificial intelligence in the workplace and the next generation of data professionals enabling business leaders to leverage data, tools, and analytical models to transform their businesses. So with that, Carrie, we’re so happy to have you here today. I’ll go ahead and turn it over to you.
Carrie Beam, MSBA Director of Analytics Projects
Good morning. Thank you so much for the invitation. I'm going to share my screen. Are you all able to see this here? Dave, can you give me a nod if you can see it?
Dave Cowan
Two thumbs up.
Carrie Beam
Fantastic. Well, thank you again, all. I am so delighted to be here. The only thing better than AI on a Thursday morning is AI with all of you on a Thursday morning.
Today, we're going to talk about capturing competitive advantage with AI, unlocking the power of AI for your business. Here’s an outline of today’s talk. We’ll talk a little bit about me, and then we’ll talk more about AI—how it works, what its strengths are, and what its weaknesses are. I’ll present some research on which job types will be most impacted by AI—some will get a boost, while others may experience a drag.
Next, I’ll cover how to evaluate a statement of work when hiring analytics professional services. Many of you don’t do it yourself but hire it out. Here’s a checklist of things to look for and reasons why analytics projects fail. Lastly, I’ll discuss how to partner with UC Davis for a student practicum capstone. We call this the “Easy-Bake Oven” version of analytics—you don’t actually have to do it; we do it for you, and then we serve it up on a silver platter with a little blue-and-gold Aggie ribbon on top.
Thank you again for the introduction. This is me looking all professional—I studied at Princeton back in the day.
This kind of thing is not going to be creatively becoming the next Picasso yet. So ChatGPT, what is it? A whole bunch of you sometimes get this alphabet salad on the end of it backwards. I'm going to help you remember: the G goes first, then the P, and then the T. So GPT stands for Generative Pre-Trained Transformer.
Generative means it makes new patterns. Pre-trained means it uses previous patterns as its training set. And then a transformer changes inputs to outputs, so it changes prompts into answers.
So how does it work? Here's a very simplified explanation from Stephen Wolfram. It scans large amounts of training data and compiles statistical predictions about what word comes next. For example, in Wolfram's paper, the sentence is: “The best thing about AI is its ability to…” The model looks at the entire internet and decides that the word "learn" happens 4.5% of the time. So most of the time, it'll choose the most popular answer. But if it always chose the most popular answer, it would be very flat and the same. So sometimes it randomizes—about 10% of the time, it’ll choose from a top few answers.
Sometimes it can be very wrong, and we call these hallucinations. For example, if it said, "The best thing about AI is its ability to bake," that would be wrong. If you’re a subject matter expert, you would know this is incorrect, but otherwise, it would sound grammatically fine.
I think of ChatGPT like a teenager—the rallying cry of today’s youth is "sometimes wrong, never in doubt." It scans large amounts of training data, compiling statistical predictions about what comes next. A simple version predicts the next word, while more complex versions use a vector-embedded database to predict what idea comes next. So, for example, if we’re talking about pets, it could talk about dogs or cats. And it can sound extremely confident even when it's completely wrong.
What is it good at? It’s really good if you want to get the average of the internet. This idea of "the wisdom of crowds," as explained in Sir Wiki's book, works for things like the best chocolate chip cookie recipe, best interview questions, or what to pack on a vacation. If averaging opinions or errors is useful, this is your tool. It’s also great if you already have an expert who can verify its output. For example, if you need it to draft a report that you’ll proofread, this is a great use case.
What is it bad at? It’s bad with edge cases—situations where you're an outlier. For example, forecasting methods when major discontinuities exist, like during COVID-19 and its impact on supply chains. Or if you’re looking for the best tourist activities in Zurich, but you’re not an average tourist—you don’t like history, walking, chocolate, or the zoo. It’s also not great for completely new use cases, like predicting if Gen Z will purchase your new app. There’s not enough data on that yet.
What is it really bad at? It struggles with complicated cases where the expert knowledge required exceeds its current logical capabilities. A basic example of this is sequential decision analytics. In school, we call this the "beer game." The way it works is you place an order, the order is brewed at the factory, sent to a distributor, and then to a retailer, which takes a few weeks. Customer demand and inventory levels change over time. You can see this in Warren Powell’s book, where they’ve already written Python code to solve it. Computers can solve it, but AI can’t yet because it doesn’t grasp the sequential, time-dependent aspects.
Even somewhat simple cases can completely "melt its brain." For example, I asked it for a linear programming formulation to assign professors to classes—something I do all the time, so I know it works. ChatGPT responded, “I will minimize the mismatch, subject to: each class must be taught by one professor, each professor has a maximum number of classes, and each professor must be qualified to teach the class.” The result looked beautiful with Greek letters and subscripts.
Then I said, “Great, give me Python code to implement that,” and it worked. You can put it into a Python environment, and it will run. However, I asked it, “Chat, what constraints did that formulation overlook?” And it told me: we forgot time slot conflicts, workload balance, preferences, and even to ensure that 100 students could actually fit in the classroom.
I didn’t take into account seniority. So this is a good way to debug your chat outputs—ask it, “What did you overlook?” After a while, you might get a bit grumpy, thinking, “If you knew you were going to overlook it, why didn’t you include it in the first place?” The answer is simple: it’s not that smart yet.
Now, if that’s bad enough, what is ChatGPT really bad at? Keeping things secret. Here's an example straight from chat. It explains that its language models use data that users provide, and those users are people like you.
If you go on Reddit and ask, “Should I let ChatGPT proofread a top-secret document?” you’ll get some warnings. Samsung’s staff uploaded super-secret information, and as a result, confidential data could have been compromised. If you’re Samsung, locked in a proxy war with Apple over patents, the last thing you want is your secrets getting mixed into a language model’s responses. So, keeping highly confidential information private is a serious issue.
Next, let’s discuss which job types are most impacted by AI. Where’s the boost, and where’s the drag? OpenAI, the same company behind ChatGPT, published a paper in collaboration with a researcher from the University of Pennsylvania. The paper explores how large language models like GPT could impact jobs and the economy. They define a concept called "exposure," which is the potential economic impact of GPT technologies on jobs.
Tasks exposed to GPT include two categories: labor-augmenting (making workers more productive) and labor-displacing (replacing workers). They describe three levels of exposure:
- No exposure: GPT doesn’t reduce task completion time, or it degrades task quality—meaning it’s not helpful at all.
- Direct exposure: Using GPT can reduce the time for a human to complete the task by 50% or more. This means workers can do their jobs faster with just the language model.
- LLM+ exposed: GPT alone doesn’t help, but when combined with other tools like image recognition or sound processing, it can reduce time to complete tasks by 50% or more.
A summary is that:
- No exposure means you're in the clear.
- Direct exposure is happening now, with tools readily available.
- LLM+ exposed requires extra software investment, but those tools are also out there.
The paper breaks down jobs into five zones:
- Zone 1: Jobs that require very little preparation (high school education or a GED). Only about 3% of these are exposed to GPT.
- Zone 2: Jobs requiring a high school diploma (7% exposed).
- Zone 3: Vocational training or an associate’s degree (11% exposed).
- Zone 4: Bachelor’s degree jobs (23% exposed).
- Zone 5: Master’s degree or higher (23% exposed).
But here’s the interesting part: if you combine GPT with additional tools like image recognition, around 75% of tasks for workers with a bachelor’s degree or higher are predicted to be impacted. So, if your business employs people in zones four and five, you need to pay attention to GPT models.
People often ask, “Is anything safe from AI?” The answer is yes. Stonemasons are safe with zero impact. Athletes and sports competitors are also in the clear—if you’ve watched the Olympics or Paralympics, very little AI involvement there. And my personal favorite: bartender helpers. Somehow the bartender role is affected, but not the helper! I thought, “Okay, maybe I’ll demote myself to helper.”
In summary, nearly all knowledge-based jobs will be impacted by AI, though some more than others. The impact doesn’t have to be negative. In many cases, GPT will augment jobs and speed up tasks, rather than replace workers. Jobs requiring a college degree are expected to be the most affected, especially by GPT and advanced LLM technologies. This will be even more pronounced in the next five years as these tools become more affordable, moving from million-dollar projects to $20-a-month subscriptions.
People often ask, “I’ve heard AI is good at some things but not others. How can we tell the difference?” Well, our friends at the Boston Consulting Group, in collaboration with Harvard Business School, had the same question. They conducted a study and wrote a paper titled "Navigating the Jagged Technological Frontier", which examines the impact of AI on knowledge workers.
They define the "jagged technological frontier" as tasks that are within the frontier (meaning they can be easily done by or with AI) and tasks outside the frontier (meaning they’re not easily accomplished by AI). That concept is simple enough, but how do we actually tell what’s inside or outside the frontier? It’s more complicated than it looks.
Let’s try an example with crowd participation. Imagine two scenarios. On the left, you’re working for a footwear company to develop new products. You need to generate ideas for a new shoe, pick the best idea, make a prototype, come up with a list of steps, name the product, and segment the footwear industry. On the right, Harold Van Mulders wants you to help pick one brand to focus on. You need to support your views with data and interview quotes, and propose innovative, tactical actions.
Now, which task do you think is easier for AI to handle—Task 1 (left) or Task 2 (right)? Go ahead, drop your guess in the chat.
All right, it looks like most of you voted for the left task, and you're correct! Task 1 is within the AI frontier, and it’s easier for AI to handle. Why? Because the internet average is a great proxy for this kind of task—brainstorming, coming up with new shoe ideas, product names, or launching steps. There’s a wealth of data out there, and AI can process it efficiently without the need to resolve conflicting internal data.
But what about Task 2? AI struggles here because it’s outside the frontier. In this case, the internet average isn’t helpful. The task requires specific, internal company data—data that’s unique to the organization. Plus, AI is poor at reconciling conflicting information, like when interview quotes don’t match up with Excel data. This is where human analysts excel because they can ask questions and dig deeper. AI, on the other hand, gets stuck.
So, is AI always helpful? If a task is within the AI frontier, humans can do the work faster and better, and employees who are less skilled at the task get the biggest performance boost. However, for tasks outside the frontier, AI may make humans faster, but the quality of their work often declines.
Now, let’s talk about how useful AI can be. We asked our friends at Amazon, specifically Andy Jassy, about their AI assistant. Here’s a quick quiz for you: How much money do you think Amazon saved by using their AI refactoring assistant? Was it $10 million, $130 million, or $260 million? I’ll give you a hint—Jassy said it was a game changer.
If you guessed $260 million, you’re right! Amazon saved $260 million and the equivalent of 4,500 developer years of work by using AI to refactor their code. That’s a huge amount of labor they didn’t need to hire for.
So, will AI eat your job? My answer is no—but people in your field who are also trained in AI probably will. What should you do? You can upskill. If you come to the UC Davis Graduate School of Management, we offer a part-time MSBA program that allows you to keep working while gaining valuable AI skills. It’s a two-year program, with a tuition discount for working students, and classes in downtown San Francisco on Thursday nights, Fridays, and Saturdays.
AI and machine learning are integrated into the curriculum, and when students finish, your employees are not only still with you, but they’ve become experts in your field—whether that’s accounting, marketing, or manufacturing—plus they now understand AI. We are number one worldwide for return on investment. And you’ll get to work with fun students who metaphorically fly on blue clouds over the Golden Gate Bridge! If you’re interested in offering this program to your employees, please reach out to me.
Now, on to our next topic: how do you evaluate a statement of work (SOW) when hiring analytics professional services? Most standard SOWs include typical items such as an executive summary, objectives, background, deliverables, requirements, and acceptance criteria. For analytics projects, however, there are a few must-have additional items:
- Technical Project Objectives – These need to be clear and specific to analytics.
- Intellectual Property Ownership – You must define ownership not only of the data but also of the algorithms developed. Often, your contract will state that while you retain ownership of your data, the service provider may use public algorithms and reuse them for other clients. Make sure this is clear in the SOW.
- Data Privacy and Security – You need to plan for what happens in case of a data breach. Back up your data and test those backups regularly.
- Coding Standards and Version Control – Ensure that version control (e.g., GitHub) is part of the plan, as well as a protocol for backing up code. If a system fails, you need to be able to restore everything quickly.
- Software Choices – Decide upfront whether to use open-source options like Python or R (which don’t require licensing fees) or commercial software (which often comes with ongoing costs).
- Data Assessment – If you’re unsure of your data, this could be step one. A data assessment determines what data you have and what’s in it. If you don't have this assessment, the SOW will typically state that the provider will conduct one before proceeding.
Now, let’s discuss why analytics projects can fail. It’s not always just bugs or backup issues—failures can be costly. Take Zillow, for example. They ended their Zillow Offers program, which used automated pricing and purchasing algorithms, after incurring a $550 million loss. The issue? The algorithm performed well in 2020, but by 2021, unforeseen changes in material and labor costs threw off the model's forecasts. They ultimately laid off 20% of their staff. This highlights what happens when models fail to adapt to significant market shifts.
Let’s go over some common mistakes in model building. These are the kinds of things you want to discuss with your analytics contractor:
- Testing the Model on Training Data – If you only test your model on the training data, it will look great—but in the real world, it won’t perform well. You need to test it on separate, unseen data.
- Allowing Target Leakage – For example, if you’re predicting house prices, you don’t want the real estate agent’s estimate of the price to be part of the model, because in the real world, you won’t have that expert opinion available when making predictions.
- Using Vanilla Forecasting Methods during Big Discontinuities – If major changes are happening, such as shifts in material costs or labor markets, you can’t rely on standard statistical forecasting methods.
- Overfitting or Underfitting the Model – Overfitting means the model is too complex, while underfitting means it’s too simple. You need a balance, getting the model just right.
- Using Fancy Models with Poor Explainability – If you can’t explain why the model recommends something, that’s a problem. You want clear reasoning, like “People who buy peanut butter often buy this type of jam—would you like to add it to your cart?”
- Not Testing Recommendations Thoroughly – Many don’t test the outcomes of their model’s recommendations sufficiently, which can lead to issues down the line.
By avoiding these common mistakes and ensuring your SOW covers all the necessary bases, you can increase the likelihood of success in your analytics projects.
If you're rolling out any kind of automated machine learning in a production setting, it’s critical to test it first—either with a single customer or, even better, run a simulation under a variety of conditions on your computer before moving expensive real-world products. Now, I’d like to share how you can partner with UC Davis for a student practicum capstone. This is like the Easy Bake Oven of analytics!
Let me introduce two of our partners: Standard Insights and Broad Vision Marketing. Jerry from Standard Insights and Jaco from Broad Vision Marketing are here with us today, and both partnered with us last year. With their permission, I’m going to share some of the exciting student projects they worked on.
First, let’s talk about Standard Insights. This company provides AI as a service, taking raw first-party data (e.g., grocery store checkout data) and turning it into valuable insights to target the right person with the right product at the right time. They’re not just selling products—they’re selling the knowledge of who buys those products and when.
Our students—Avani Hitesh, Victor Yao, Meghana, Hanwei Yao, and Victor Mao—worked on a project aimed at maximizing strategic customer engagement and revenue. They computed key metrics, normalized the data, and ran a k-means clustering model to find the optimal number of customer segments.
Here’s what they found: for example, cluster one (shown in dark green) represents your high-value customers, while cluster five (in red) are customers who might be on the verge of disengaging. With this analysis, Standard Insights can take action to re-engage those red cluster customers before they disappear.
Now, let’s look at Broad Vision Marketing. The students—Ava Chen, Nima Guha, Cindy Jian, Charles Wang, and Jizhou—focused on digital marketing for this HubSpot-certified company, which serves industries like healthcare and home building. Their problem statement was simple: How can we help clients rank higher on Google?
For this project, the students focused on the pharmaceutical industry and built a web scraper to collect data on high-ranking websites. They then applied multinomial logistic regression and random forest machine learning methods.
The results were clear: websites on pages 1 to 5 of Google typically have 5 to 7 videos, while websites on pages 21 to 25 had only 0 to 1 video. They also found that word count doesn’t matter as much in this space, but videos and call-to-action links are crucial for higher rankings.
This analysis helped Broad Vision Marketing’s clients optimize their web content, improving their chances of ranking on the first page of Google and potentially increasing click-through rates by 1-5%.
In summary, the UC Davis MSBA program offers opportunities to work with young, talented students on impactful projects. We have around 100 students, the program is one year long, and it's based in downtown San Francisco. If you’re interested in partnering with us for a student practicum capstone, please feel free to reach out!
When you work with us on a project, you'll have a team of four to six students for about 10 months, with each student contributing around eight hours per week. You'll meet with them weekly, and they'll solve real business problems. These problems could be related to marketing mix, product design, volunteer engagement, bundling, and more. Many companies are also asking for chatbots and customer targeting solutions. Additionally, we offer database refactoring, which we call, "I made a mess of my data and I'd like you to clean it up." One of our partners currently has 100 million rows of public-facing data we're working through.
So, why do industry partners love working with us? Here’s why:
- Embedded, sustained workforce support: We don’t just finish a project and leave—we continue to provide cost-effective resources.
- Access to fresh talent: You get students trained in the latest analytics tools and techniques, ready to bring new ideas and perspectives.
- Networking and collaboration opportunities: We provide great opportunities to connect with a variety of industries.
- Recruitment pipeline: You'll get first access to our students' resumes and portfolios.
- Faculty support: If you face a particularly challenging problem, UC Davis faculty will provide additional expertise.
To recap today's talk, we covered:
- AI: Its strengths and weaknesses. AI performs best when you’re seeking results close to the average.
- Job impact: AI will most affect jobs requiring higher education, especially those within the technological frontier. CPAs, you’re in the crosshairs!
- Statement of Work: It’s essential to clearly define ownership of data and models and ensure a thorough data assessment is part of the process.
- Analytics project failures: There are plenty of ways to fail, but avoiding the six biggest mistakes in analytics projects will increase your chances of success.
- Partnering with UC Davis for student capstone projects: It's easy! Just email me at cbeam@ucdavis.edu, and we’ll get started.
Finally, feel free to screenshot anything you’d like. For more detailed reading, I encourage you to capture this slide with links to research papers and a case study about Amazon saving significant costs through data analysis.
With that, I’ll stop sharing and open the floor for questions. Thank you so much!
Dave Cowan
Thank you, Carrie, for the fantastic presentation. As a reminder to the audience, we will post a recording of today’s talk on the Modern Ideas for Business website, along with a copy of Carrie’s presentation. Those should be available within 24 hours.
Now, let’s start the Q&A. If you'd like to ask Carrie a question, please use the "Raise Hand" feature, and we’ll unmute you.
Jerry Avyog, Standard Insights
Hi, this is Jerry from Standard Insights. We had the privilege of partnering with Carrie and her students last year and just had another meeting earlier today for our second project. I want to mention a couple of things we learned that have greatly benefited Standard Insights.
First, working with UC Davis has built up our credibility. Partnering with a top-tier analytics institution, located in the Bay Area, instantly gives us that credibility, especially as AI is becoming an overused buzzword.
Second, some of the insights Carrie shared earlier—like RFM analysis—we’re actively implementing as part of our marketing materials for an upcoming trade show in Miami for the food and beverage distribution industry. It’s been a tremendous benefit to work with UC Davis students.
I’d like to add that our partner companies, sponsored by Zebra Technologies, a billion-dollar OEM manufacturer, are seeing real-world benefits from AI. What Carrie mentioned about AI's future implications is critical, and it aligns with what we’re experiencing.
Jaco Grobbelaar, Broad Vision Marketing
I also have more of a statement. One of the greatest benefits of working with Carrie and her team was the clarity they brought to our project. Initially, we struggled to clearly define the problem, but within five minutes of Carrie joining a meeting, she whipped us into shape with her experience. That made a huge difference for the project outcome.
I remember feedback from a UC Davis pharmacist who had worked with the program for four years. He mentioned that each year he refined his ability to define the project better, which led to improved outcomes. This is our second year working with UC Davis and Carrie, and we’re much more precise in thinking through what we want to achieve.
One tangible benefit we’ve seen is in our data analysis. Initially, it took us 8-10 hours to analyze a website for optimization. Now, after working with Carrie’s team, we can complete the same analysis in one to one-and-a-half hours. That’s a significant gain in efficiency for us
Ed Correia, Sagacent Technologies
Thank you, Jaco. David, I see you have a question for Carrie.
Dave Cowan
Carrie, there’s a lot of interest from participants in your program. Could you talk about how you decide which projects to work on? I assume companies approach you with ideas. How do you determine which projects will benefit from your team's efforts?
Carrie Beam
Great question, David. The first step is always a conversation with me. We start by asking, Is there a business problem? Then, Is there data available? Sometimes the company has internal data, like Standard Insights, or they may use external data, like with Broad Vision Marketing, where we scraped Google’s data. I help them identify the data sources and algorithms that might be appropriate.
Next, we assess if the company can commit to an hour a week to meet with the students and whether they’d provide a good partnership experience. We work with companies of all sizes—from single-person startups to Fortune 500 organizations. We typically prefer them to be incorporated, either as LLCs or otherwise, and provide solid data and a positive student experience.
Sometimes it’s not the right fit immediately, but I guide them on steps to take, like preparing their data better, so they can participate in future years
Ed Correia
Thanks, Carrie. While we wait for more questions, I’d love to hear your thoughts on something else. People often lump AI, automation, and robotics into the same category. I appreciated your explanation of AI’s impact on job sectors, but could you comment on the differences between AI and automation/robotics? I see the latter as more of a threat to lower-skilled jobs, while AI seems to target higher-education roles. As a farmer’s son, I’ve seen how machinery impacted farming, and I imagine autonomous vehicles might do something similar for other industries.
Carrie Beam
Absolutely, great point! AI, like text-based systems (e.g., GPT), tends to impact college-educated jobs—those roles where people spend their time typing and producing content or analysis. It’s much easier to apply AI to these tasks because it involves repetitive, cognitive work that AI can mimic.
On the other hand, automation and robotics are more physical. It’s much harder to replace jobs involving movement and manual labor with robots. While advancements are happening, especially with autonomous vehicles, jobs that require complex physical manipulation are harder to automate. So, yes, I agree—they present two different kinds of threats: AI is more focused on higher-education jobs, while automation and robotics threaten the lower-skilled, manual workforce.
Carrie Beam
It's incredibly difficult to teach a robot to pick up a cup. Teaching an autonomous vehicle is equally challenging, and that's why CAPTCHA tests ask us to identify traffic lights and sidewalks—they're gathering training data. It's still not quite as good as it needs to be. The challenge comes from integrating computer vision and computer motion fast enough in 3D environments with motion, like picking things up or driving. The technology will impact certain jobs, but it's harder to replace these tasks than it looks.
Ed Correia
Thanks, Carrie. Dave, I see you have a comment.
Dave Cowan
Yes, I just want to make a point. Many on this call might wonder how to apply this to their own work. One interesting insight came from Jaco. He realized you don’t need data analytics specifically for your company to benefit. Broad Vision Marketing thought about research Carrie and her team could do that would benefit their clients.
So, for anyone wondering whether a project can help you, consider how your clients' needs might benefit from research that Carrie’s team could do, making you more valuable as a trusted advisor.
Ed Correia
Great point, Dave. Carrie, I have another question. My son works as a paralegal for an immigration law firm, and he's been using ChatGPT for research. I wonder if you've done any work with law firms? If not, how do you see this technology benefiting them?
Carrie Beam
We haven’t yet completed a project with a law firm, but we're in talks with a few. One example, without breaching confidentiality, is with an employment law firm. They're interested in using GPT-like technology to analyze email databases. The goal is to quickly assemble emails that might support or refute an allegation.
For instance, if an employee claims their boss made them work more than 40 hours a week without overtime, it’s unlikely those emails would contain explicit keywords like "overtime violation." Instead, we can train GPT to look for contextual phrases, like, "Hey boss, I’m working late again," or "My paycheck is short." This allows us to flag relevant conversations without needing specific keywords.
Ed Correia
Thanks, Carrie. We’ll open it up for more questions. While waiting, I want to reiterate something for the group. Some of you might be thinking, "I already knew all of this," and in that case, you’ve earned yourself a cappuccino. Others might feel like this is information overload—and that’s okay! This happens to all of us, especially in a rapidly evolving field like AI.
Carrie Beam
I encourage you to use the tools available. Whether it’s worth paying for premium versions of AI tools (at $15-$20 a month), try incorporating them into your daily life. Of course, avoid uploading confidential business information, but use these tools to practice.
For instance, if you’re trying to decide what to wear to a business meeting, ask it for suggestions. Or if you want to know the macronutrient content of your lunch, input what you're eating. Using these tools frequently builds fluency, similar to learning a new language.
Lastly, find someone from Gen Z—ideally a middle schooler. They have no memory of a world without instant connectivity, social media, or AI. Their fluency with these tools is second nature, and they can provide valuable insights on how to better integrate AI into your work or personal life.
Participant
It's interesting that your first example applies to me right now because we're leaving for South Africa and Europe in about 15 days. We're figuring out what to do, and the framework you mentioned really helps narrow things down.
Carrie Beam
Absolutely! You can also debug those plans by asking questions like, "What are some things we should do on this trip?" And then ask, "What did that list forget?" or even, "If something went horribly wrong, what would it likely be, and how could I have prevented it?" It helps you refine your plans.
Participant
That's an interesting idea—thanks! By the way, we've identified the person who had a question earlier. Her name is Carrie—Carrie, would you like to ask?
Jennifer
Hi, it's actually Jennifer, but I go by Carrie sometimes! Thank you for this fabulous session. I'm currently based in Oslo, Norway, and I do a lot of speaking about AI and its integration into society and business. One of the big questions here is around regulations. What's your point of view on whether the government or corporations should be responsible for regulating AI to ensure its safe use?
Carrie Beam
Great question! I think the answer is culturally driven. For example, with GDPR, the European approach tends to prioritize privacy and citizen safety, even if that means corporations make a little less profit. In contrast, in America, we often prioritize corporate profit, sometimes at the expense of privacy and safety. So, regulations will likely depend on the cultural values of where you are.
Host
Thanks, Carrie! Any final questions before we close?
Carrie Beam
Feel free to reach out! I’ll drop my email in the chat if anyone wants to talk further—whether it's about guest speaking, working with our students, upskilling your employees through a part-time MSBA, or discussing a project for next year. I’d love to chat!
Ed Correia
Thank you all for attending today's webinar, and a special thanks to Carrie for a fantastic presentation. We'll email a copy of the presentation to everyone, and it will also be available on the Modern Ideas for Business website.
Next month, we have a webinar on October 10th with Nicole McKenzie, founder and CEO of Momentum Accounting, who will discuss the secret to sustainable profitability. In November, Clint Tripati will talk about making strategy your next best habit.
If you'd like to be added to our mailing list, drop your email in the chat. Our goal for 2025 is to host a webinar every month, so stay tuned for more events.
Thanks again, and have a great day!